The state-of-the-art in trust research provides roboticists with a large trove of tools to develop trustworthy robots. However, challenges remain when it comes to trust in real-world human-robot interaction (HRI) settings: there exist outstanding issues in trust measurement, guarantees on robot behavior (e.g., with respect to user privacy), and handling rich multidimensional data.
We survey recent advances in the development of trustworthy robots, highlight contemporary challenges, and finally examine how modern tools from psychometrics, formal verification, robot ethics, and deep learning can provide resolution to many of these longstanding problems. Just as how advances in engineering have brought us to the cusp of a robotic revolution, we set out to examine if recent methodological breakthroughs can similarly aid us in answering a fundamentally human question: _do we trust robots to live among us and, if not, can we create robots that are able to gain our trust?
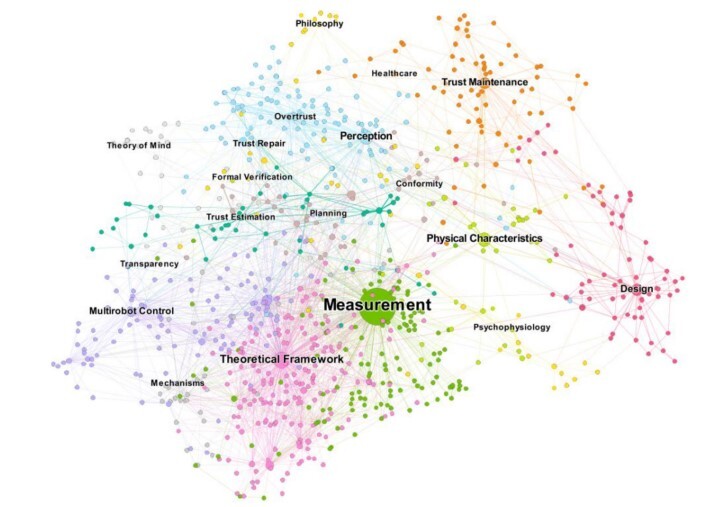
Fig 1. An overview of trust research in robotics.Each node represents a publication, whose size scales with citation count. Initial work concentrated heavily on measuring trust in automation/robots (green node in the middle). Since then, research in the area has branched out to examine areas such as the multirobot control and the design of robots, as well as new theoretical frameworks for understanding trust in robots. The most recent work has explored novel topics such as formal verification in HRI
First, built-off previous work[1,2,3,4,5], we take an utilitarian approach to defining trust for HRI. We define an agent’s trust in another agent as a:
“multidimensional latent variable that mediates the relationship between events in the past and the former agent’s subsequent choice of relying on the latter in an uncertain environment.”
How can robots modulate user trust?
We survey prior works that address gaining, maintaining and calibrating user’s trust in robots, and find the following four major groups of strategies:
- Design: Physical Appearance of a robot can bias humans towards trusting or mistrusting it.
- Heuristics: Rule-of-thumb responses, often inspired by emperical evidence from psychology, to combat overtrust and to repair trust.
- Exploiting the Process: Mediating how a robot behaves (for e.g. w.r.t transparency, vulnerability, risk-averseness etc.) can affect the user’s trust.
- Computational Trust Models: The above methods rely mainly on pre-programmed strategies. In contrast computational trust models explicitly model the human’s trust (often as a latent variable) and use planning to take actions that modulate the trust.
- Robots with Theory of Mind: Endowing robots with ToM, gives them the ability to reason about the user’s mental attributes, beliefs, desires and intentions and respond accordingly, thus implicitly effecting the user’s trust in the robot.
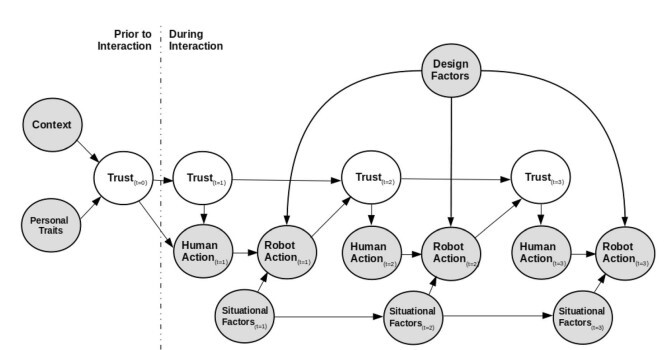
Fig 2. A typical PGM representation of a computational trust model that represents the user's trust on the robot as a latent variable.
Challenges and Opportunities
We highlight three key challenges and the corresponding opportunities in trust research that could be the focus of much inquiry in the coming decade:
- The Measurement of Trust “In the Wild”: Current trust measures lack confirmation testing, addressing measurement invariance and information regarding psychometric properties of objective measures.
- Bridging Trustworthy Robots and Human Trust: Few works have explored topics such as the utility of applying formal methods in conjunction with human trust in robots, addressing scaling up of existing tools to real-world HRI, the affect of privacy on trust etc.
- Rich Trust Models for Real-World Scenarios: There is value in taking multidimensionality seriously and devloping richer and more accurate models for the antecedents of trust. Additionally, richer models can also be created by leveraging the power of deep probabilistic models that can deal with high-dimensional real-world data.
Citation
If you find the ideas presented in our paper useful for your research, consider citing our paper. Kok, Bing Cai, and Harold Soh. 2020. “Trust in Robots: Challenges and Opportunities.” Current Robotics Reports 1(4): 297–309.
@article{Kok_Soh_2020,
title={Trust in Robots: Challenges and Opportunities}, volume={1}, ISSN={2662-4087}, DOI={10.1007/s43154-020-00029-y}, abstractNote={To assess the state-of-the-art in research on trust in robots and to examine if recent methodological advances can aid in the development of trustworthy robots.}, number={4}, journal={Current Robotics Reports}, author={Kok, Bing Cai and Soh, Harold}, year={2020}, month={Dec}, pages={297–309}, language={en} }
Contact
If you have questions or comments, please contact Harold Soh.
References
[1] Mayer RC, Davis JH, Schoorman FD. An integrative model of organization trust. Academy of Management Review. 1995;20(3). https://doi.org/10.5465/amr.1995.9508080335
[2] Hoff KA, Bashir M. Trust in automation: Integrating empirical evidence on factors that influence trust. Human Factors. 2015;57(3). https://doi.org/10.1177/0018720814547570
[3] Lewis, M., Sycara, K., Walker, P.: The role of trust in humanrobot interaction. In: Studies in Systems, Decision and Control, vol. 117, pp. 135–159. Springer International Publishing (2018). DOI https://doi.org/10.1007/978-3-319-64816-3\8
[4] Lee JD, See KA. Trust in automation: designing for appropriate reliance. Human Factors: The Journal of the Human Factors and Ergonomics Society. 2004;46(1):50–80
[5] Castelfranchi, C., Falcone, R.: Trust theory (2007).